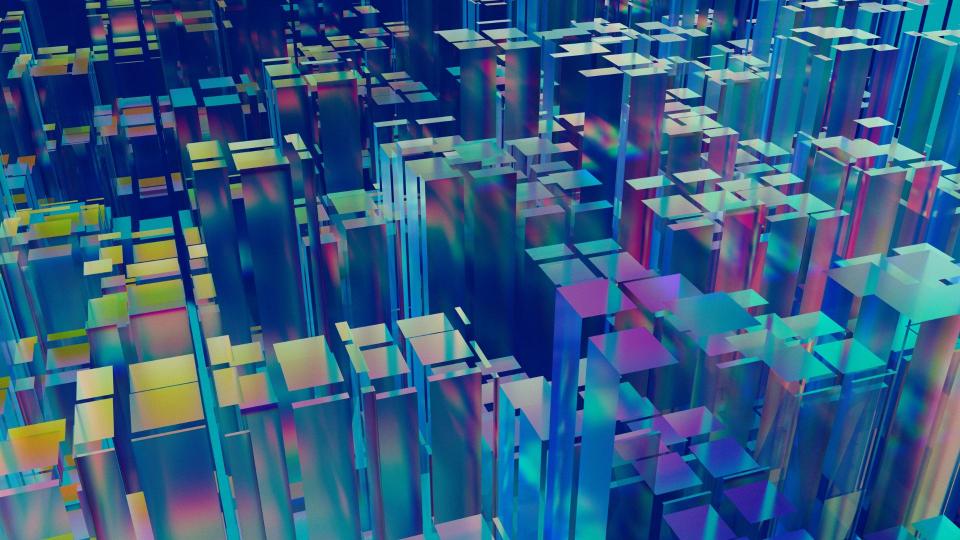
Photo by and machines on Unsplash
In August 2023, I made a peculiar observation while searching for information about late Zimbabwean writer, Yvonne Vera. The first Google image result accompanying information about her was actually not an image of Vera. Rather, the photograph was of another renowned Zimbabwean writer, Tsitsi Dangarembga.
I wanted to believe it was some sort of technological glitch; perhaps the algorithm was out of whack that day (really, I should know better by now than to invest that sort of faith in technology). But upon running the same search again a few weeks later, it yielded the very same results. I posted about this on Twitter and LinkedIn and it would seem some of my connections sounded the alarm with Google. This led to the matter being resolved in under 24 hours; by the next day, the 4th of August 2023, a search for Yvonne Vera accurately returned a main image result of her.
The image of Dangarembga still, however, appeared among the general results for Vera. Since Google returns its results by crawling the internet (websites and internet pages) and searching the metadata of images, my guess is that the photograph of Dangarembga – which had showed up as the primary result for Vera – had initially been miscredited as an image of Vera at the source of publication. This points to human error which was then rectified, but which continued to have consequences on search results for Vera as the Google search engine algorithm continued to promote the page (and the image it carried) with the original error. I am not sure how long this image result of Dangarembga had been showing up as a result for Vera, but we know that Google’s cache can last for any time up to 90 days.
We also know that technology is not perfect; it is, after all, trained by human beings who are by no means perfect themselves. And algorithmic bias comes into technology in many ways, including by way of who builds the technology, who uses it and how. In the book ‘Algorithms of Oppression: How Search Engines Reinforce Racism’, Safiya Noble observes that:
“Part of the challenge of understanding algorithmic oppression is to understand that mathematical formulations to drive automated decisions are made by human beings.”
Noble further adds that:
“While we often think of terms such as “big data” and “algorithms” as being benign, neutral, or objective, they are anything but. The people who make these decisions hold all types of values, many of which openly promote racism, sexism, and false notions of meritocracy, which is well documented in studies of Silicon Valley and other tech corridors.”
Algorithmic bias comes into technology in many ways, including by way of who builds the technology, who uses it and how.
For years, human bias has translated into technological bias in many ways. Until quite recently, for example, Google searches for terms like ‘black girls’ yielded results that stereotyped them alongside other often hypersexualising terminology.
It, therefore, becomes important to think through whether this Vera/ Dangarembga mix up was indeed an error; or if it actually emanated from a similar bias. Perhaps the person inputting the metadata into the image didn’t know the difference between the two women. The image is from a Zimbabwean website, but given the diminished literary culture in the nation and continued marginalisation of women’s cultural contributions, it is safe to assume that a level of bias (conscious or unconscious) played a role in the error. Error can be informed by bias; a feeling of a lack of need to verify if an image of one Zimbabwean woman author is not that of another because “how many of them can there possibly be?” and “surely, this is that same Black woman writer with the dreadlocks because how many Black Zimbabwean women writers with dreadlocks are there anyway?” These are both distinguished award-winning writers known globally for their work. And if it can happen to women of such high repute, what does that mean for those with far less renown? How much more of African women’s lives is being inaccurately archived digitally? It’s that sort of lackadaisical – and often patriarchal – attitude that says if you’ve seen one of them, you have seen them all.
And this may then hold true for a lot of what we then think of as human error in algorithmic misrepresentations. Bias is informed by a range of values, ideas and beliefs, and is presented in many different ways. Too often, we don’t really unpack what informs error beyond a general idea of absent-mindedness or distractedness. Human error too is political. It is just as important for us to think through how technology can prolong, and even amplify, bias through human error. And how to go about addressing this within our non-digital realities. Alongside this is the ongoing need to think through the monopolies over knowledge that big tech companies have. If Google shows you a main image result of Tsitsi Dangarembga as Yvonne Vera, are you likely to doubt it?
Another important question to ask is just how responsive are these algorithms to correction? By 4th of August, when Google rectified the error, the image result for Dangarembga had been demoted to the 42nd result for Vera. But by the 29th of August, this same image had risen back up in the rankings to the 20th result for Vera. I wonder if this is some sort of algorithmic fluctuation, or something about the errant page’s search engine optimisation, or the Google cache at play. But whatever it is, it reinforces the thesis that human error perpetuates bias and misinformation long beyond when we think such harm might last.
Footnotes
References:
Noble, Safiya Umoja. Algorithms of Oppression: How Search Engines Reinforce Racism, New York University Press, 2018.
- 135 views
Add new comment